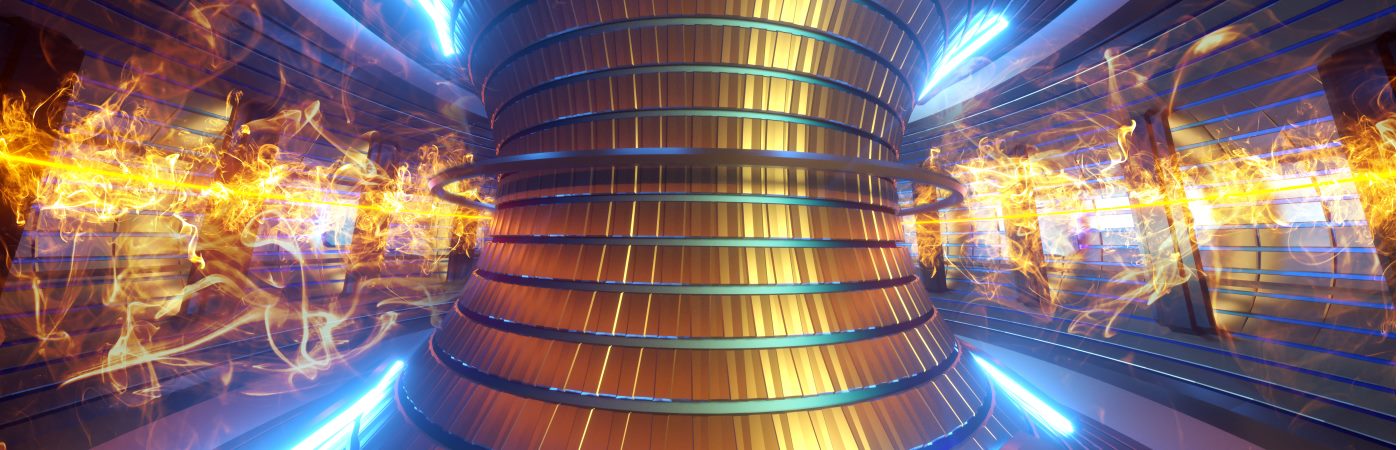
Research areas
Our research spans several key themes, aligned with UK Government research priorities, and exists to strengthen links between University researchers and the National Facilities at Harwell, including, but not limited to, Diamond Light Source, ISIS, the Central Laser Facility, and Science and Technology Facility Council’s (STFC) Scientific Computing Department.
Through our research fellows and core staff we specialise in a number of areas as described below. However we are also very open to discuss how we can support new areas of research.
-
Advanced manufacturing
How materials transform during modern manufacturing processes.
Read more
-
Advanced materials
Focusing on microscale strain and phase transformation for alloys that contains metastable crystallography structure.
Read more
-
Catalysis
Design and production of advanced novel catalytic materials.
Read more
-
Electronic structure
Identifying the organisation of electrons in matter.
Read more
-
Fusion
Carrying out research on new materials and manufacturing techniques for nuclear fusion applications.
Read more
-
Mineral physics
The properties of rocks and minerals at high pressure and temperature control the behaviour and response of the Earth over all time scales.
Read more
-
Quantum materials
Exploring the exotic order and excitations of materials at low energy scales using neutron scattering and advanced theoretical analysis.
Read more
-
Resilience
Imaging safety-critical failure of materials containing high stored energy in order to identify potential mitigation mechanisms.
Read more
-
Subsurface energy and storage
The transition of energy supply from mainly fossil sources to low carbon energy sources is essential for future environmental sustainability.
Read more
-
Transverse axis
Material response to extreme conditions is key to many fundamental and applied sciences.
Read more